Grand Challenges
Time
11:15-12:30, 11th July (Th)
Room
Auditorium 3F
Chair:
Gene Cheung, York University, Canada
Jiaying Liu, Peking University, China
Schedule:
11:15--11:20 Opening Remarks
Grand Challenge Chair
11:20--11:35 Grand Challenge: 106-p Facial Landmark Localization
-- GC Overview
Hailin Shi, AI Platform and Research, JD.com
-- Winner Talk
11:36--11:50 Grand Challenge: Learning-Based Image Inpainting
-- GC Overview
Dong Liu, University of Science and Technology of China
-- Winner Talk
11:51--12:05 Grand Challenge: Short Video Understanding Challenge
-- GC Overview
Changhu Wang, Bytedance AI Lab
-- Winner Talk
12:06--12:30 Grand Challenge: Saliency4ASD
-- GC Overview
Patrick Le Callet, University of Nantes, France
- Winner Talk for Track 1
- Winner Talk for Track 2
Time
15:30-16:30, 11th July (Th)
Room
3B
Description
As the deep learning methods have been largely developed in facial landmark localization task, the requirements of practical applications are growing fast. However, for large poses and occlusion, the accuracy of localization needs to be improved. Here, JD AI Research and NLPR, CASIA sincerely invited researchers and developers from academia and industry to participate in this competition and encourage further discussion on technical and application issues.
Website
https://facial-landmarks-localization-challenge.github.io
Organizers
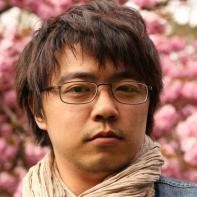
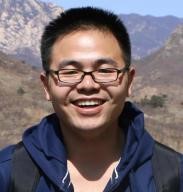
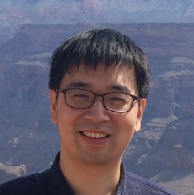
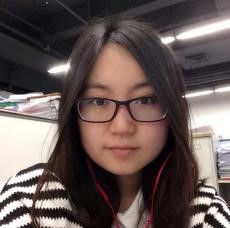
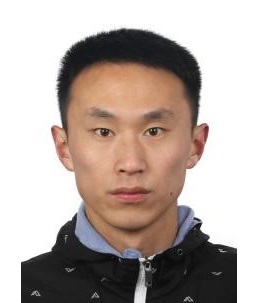
Time
16:45-17:45, 11th July (Th)
Room
3CD
Description
Image inpainting, also known as image completion, is the process of filling-in the missing areas of an incomplete image so that the completed image is visually plausible. While this task is indispensable in many applications, such as dis-occlusion, object removal, error concealment, and so on, the task is still regarded very difficult thus far. Traditionally, several different approaches have been proposed for image inpainting, including partial differential equation-based inpainting, constrained texture synthesis, structure propagation, database-assisted, and so on.
In recent years, deep learning has revolutionized the research of image inpainting, and a number of deep models have been designed. Nonetheless, the lack of a public, widely acknowledged dataset has been a significant issue in developing advanced, learning-based inpainting solution.
This challenge is meant to consolidate research efforts about image inpainting using learning, especially deep learning approach. We will prepare two tracks: error concealment (EC) and object removal (OR). In the EC track, we simulate the case of transmission error that incurs missing areas (usually square blocks) in a decoded image. In the OR track, we carefully select some objects in an image to be removed, and produce missing areas with irregular shapes. In both tracks we challenge the researchers to inpaint the incomplete image. The major difference between the two tracks is that, in the first track, we want to recover the missing areas so that the completed image is similar to the original (although this can be very difficult!), and in the second track, we are satisfied as long as the completed image is visually plausible and pleasing.
We are aware of a previous competition in conjunction with ECCV 2018, which also addresses the problem of image (and video) inpainting. Different from that competition, in our challenge we evaluate the quality of completed images by both objective metrics (PSNR, SSIM) and subjective evaluation (MOS).
Website
https://icme19inpainting.github.io/
Organizers
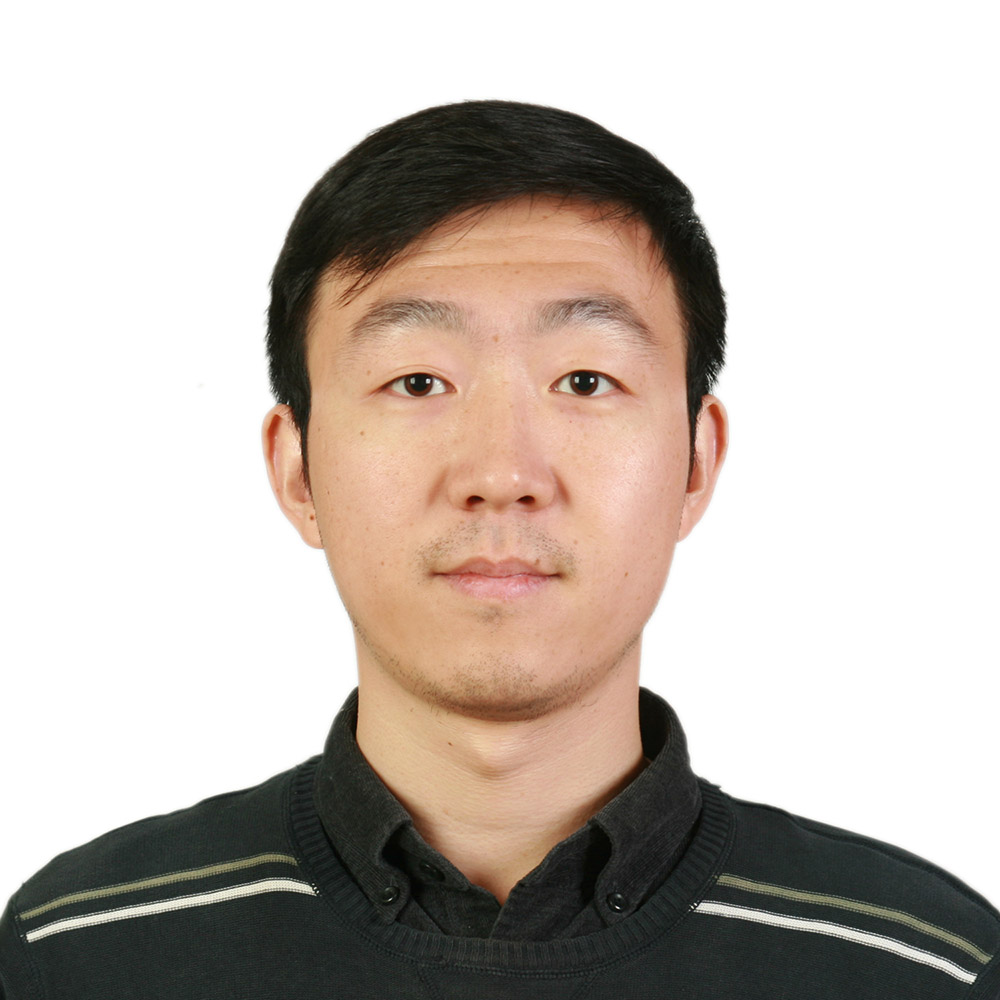
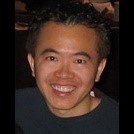
Time
14:00-15:00, 11th July (Th)
Room
3B
Description
This challenge provides multi-modal video features, including visual features, text features and audio features, as well as user interactive behavior data, such as click, like, and follow. Each participant needs to model the user's interest through a video and user interaction behavior data set, and then predict the user's click behavior on another video dataset.
Website
http://ai-lab-challenge.bytedance.com/tce/vc/
Organizers
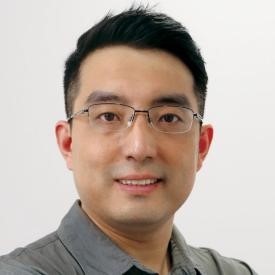
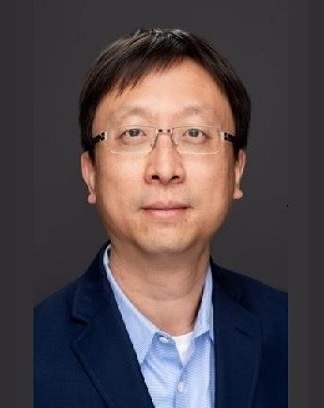
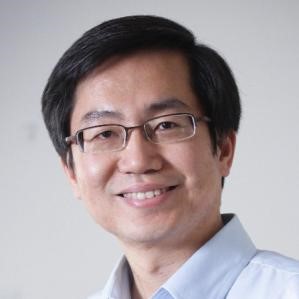
Time
16:45-17:45, 11th July (Th)
Room
3B
Description
The purpose of the Grand Challenge Saliency4ASD is to drive efforts of visual attention modeling community towards a healthcare societal challenge. Gaze features related to saccades and fixations have demonstrated their usefulness in the identification of mental states, cognitive processes and neuropathologies (Tseng et al., 2013; Itti, 2015), notably for people with ASD (Autism Spectrum Disorder).
Website
Organizers
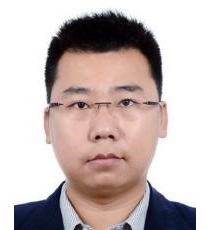
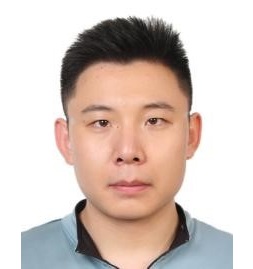
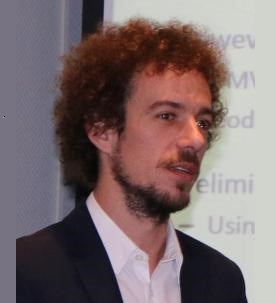
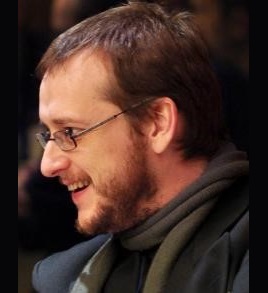
Grand Challenges Chairs
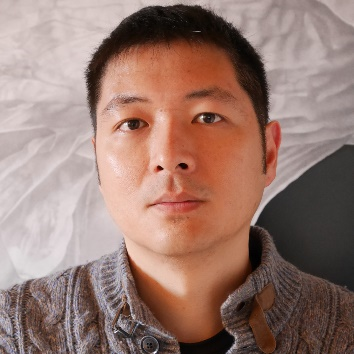
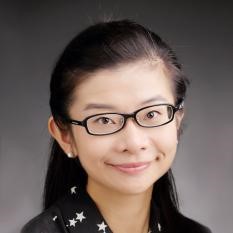